
- This event has passed.
SPE APOGCE conference preview – Use of Big Data and Machine Learning to Optimise Operational Performance and Drill Bit Design
November 17, 2020 @ 8:00 am - November 19, 2020 @ 5:00 pm
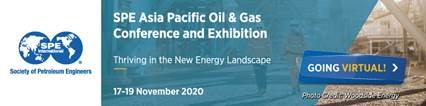
Paper Number | : | SPE-202243-MS |
Paper Title | : | Use of Big Data and Machine Learning to Optimise Operational Performance and Drill Bit Design |
Author Block | : | S. Cornel, Baker Hughes Company; G. Vazquez, Senex Energy Ltd. |
APOGCE Conference 2020 registration:
https://www.spe-events.org/apogce2020/home
Abstract
Objectives / Scope:
Time savings and bit longevity is one of the major challenges in Coal Seam Gas unconventional fields Onshore Queensland. Maximize rate of penetration based on best drilling parameter was the key target to tackle these issues. The dataset was accumulated from more than 50 bits run. A supervised machine learning algorithm was used to classify drilling parameters that increase ROP and bit endurance.
To achieve this goal, our focus was on a) optimizing PDC bit design, drilling hydraulics and b) developing a Drillers Roadmap to ensure optimal parameters were run in the field.
Methods, Procedures, Process:
Operator and service company worked closely to selecte two PDC frames and benchmark performance between them.
A novel approach was then taken to capture the rig sensors data. These steps were as follows:
Capture big data using sensors integrated on the rig to produce drilling parameters data sets.
An algorithm to clean large data sets with the use of Python Pandas libraries was created.
Using supervised machine learning and clustering the data to identify which drilling parameters gave the best results.
Create a Drillers Roadmap identify drilling parameter trends by depth and provide a “sweet spot” based on machine learning for fine tuning operational window.
Process the data set using visualisation tools to develop heatmaps vs depth for each of the drilling parameters (WOB, RPM, flow rates and Torque)
Results, Observations, Conclusions:
A formal process for optimizing performance was developed. After 6 wells optimal operating ranges were identified. This process leads the driller to respond to changes in formation and deliver more consistent drilling performance with excellent results.
ROP increased from 80m/hr to 150m/hr, driven by improved ROP in the lower section of the well
Time saving of more than 150 hrs over the drilling campaign.
Refine PDC bit designs based on optimized parameters, therefore improving bit life through cutter and hydraulic enhancements.
Novel / Additive information:
The innovation of this methodology is to gather drilling information, cleaning the data with Python using Pandas library thus making this process more efficient.
After the data files were clean and QAQC, a supervised machine Learning algorithm was used to optimize ROP’s by taking drilling datasets and fine tuning the parameters (WOB, RPM, Flow rate) using drilling dynamics (vibration) and MSE models.
Finally, innovative visualizations (heatmaps) were produced in order to give a clear insight of the drilling information gathered by the rig sensors to the company men and drillers.